My research goal is to understand the neurocomputational basis of acquiring abstract task knowledge. At a mechanistic level, how is the brain able to grasp overarching principles, patterns, and relationships that generalize across diverse behavioral contexts? For example, upon visiting a new country, one can readily generalize the check-out rules of a single restaurant to the other restaurants of that same country. Although this capacity for generalization is considered a key ingredient of intelligent behavior and a missing element in artificial neural networks to emulate human-like performance, its neural underpinning remains unknown. To address this gap, my future research program lies at the intersection of neuroscience and artificial intelligence (AI). By discovering the brain's mechanisms for acquiring abstract knowledge, we will inspire the development of new algorithms for general-purpose, flexible AI systems, which, in turn, provide deeper insights into brain function.
I have taken multidisciplinary approach that involves complex behavioral tasks, simultaneous cortex-wide imaging, electrophysiology, causal manipulations, and computational modeling. I have also incorporated methods from geometry and topology to discover a meta-level algorithmic language that can integrate computations in multiple scales of brain operation: neural activity, circuits, and behavior. This pursuit will contribute to a comprehensive and mechanistic understanding of the neural substrates underpinning abstract knowledge. Ultimately, I intend to utilize this foundational understanding as a basis for devising cutting- edge brain stimulation techniques that help alleviate symptoms of brain disorders.
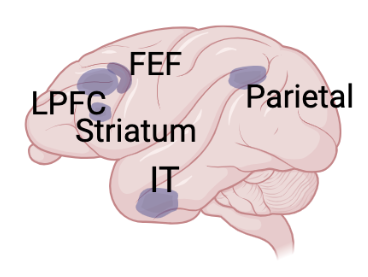
Neurocomputational basis of compositionality
Animals efficiently learn abstract task structures and later recompose this knowledge to deal with novel contexts, an ability that is called compositional generalization. Such capacity can grow their behavioral repertoire through combinatorial expansion. During my postdoc at Tim Buschman’s lab, I designed a novel behavioral paradigm where monkeys performed three compositionally-related rules. In collaboration with group of Nathaniel Daw, we built a computational model of animals’ behavior. We found that animals simultaneously combine trial and error learning and abstract structure learning strategies to perform this task. I then simultaneously recorded from fronto-parietal network, basal ganglia, and temporal cortex. I found that brain uses shared neural subspaces to compositionally build new rule representations. Moreover, these shared representations were dynamically recruited while the animal was learning each rule. Importantly, the brain warped the geometry of neural representations to recruit relevant subspace. This series of works will elucidate how the brain re-uses abstract task representations to flexibly build new representations in novel contexts.
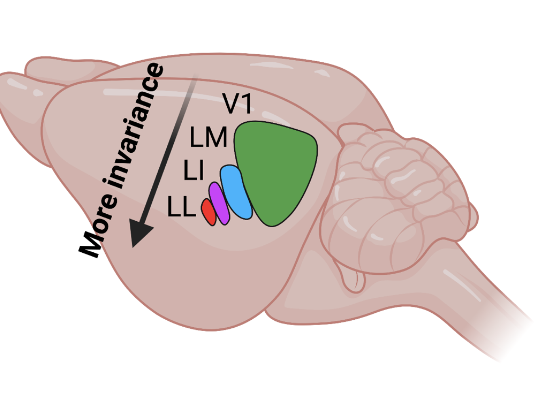
Neural, behavioral and computational basis of invariant object recognition
During my PhD in the lab of Davide Zoccolan at SISSA, Italy, I performed series of experiments to demonstrate the behavioral, neural, and computational basis of invariant object recognition in rats. Invariant object recognition is the ability to recognize objects despite variation in their appearance such as orientation and size. This allows us to distill diverse object representations into a generalized and abstract representation. As the first step, I conducted a behavioral study using priming paradigm, which demonstrated rats can perform invariant object recognition. Building upon this, I proceeded to carry out multielectrode recordings from four different visual areas (V1, LM, LI, and LL) that are analogous to the primate ventral stream. In collaboration with the theoretical group of Stefano Panzeri, we used information theory to show that neurons along this hierarchy become progressively tuned to more complex visual object attributes while also becoming more view-invariant. Moreover, we found that representation at the neural population level along the hierarchy become more linearly separable, suggesting a reformatting of representation from tangled representations in low-level areas such as V1 to untangled representations in higher-level areas such as LL. These results show how transformation of geometry of neural representations enables the emergence of abstract representations. In collaboration with Paolo Muratore, we found similar reformatting of representations in rat visual cortex and deep neural networks, Overall, the combination of my behavioral and neurophysiological studies in my PhD suggest that rodents share with primates some of the key computations that underlie the invariant object recognition and point to the rodents as promising models to dissect the neuronal circuitry of this ability.